Model Hub
The SambaStudio platform provides a repository of models available to be used for a variety of applications. You can access the repository from either the GUI (the Model Hub) or the CLI (the model list).
Base models do not support inference and cannot be deployed for endpoints. It is recommended to use Base models for training and not inference. |
This document describes how to:
View the Model Hub using the GUI
Click Models from the left menu to navigate to the Model Hub. The Model Hub provides a two-panel interface for viewing the SambaStudio repository of models.
See Create your own CoE model to learn how to create your own Composition of Experts model. ![]() Figure 1. Create CoE model
|
- Model Hub filters
-
The Model Hub filters in the left panel host a robust set of options that refine the display of the model cards panel. In addition to the selectable filter options, you can enter a term or value into the Search field to refine the model card list by that input.
- Model cards
-
In the right panel, model card previews are displayed in a three column grid layout. The tabs above the grid filter the displayed models by status:
-
All models displays every model in the Model Hub, including downloaded models, upcoming models, and models that are ready to be downloaded. These models can be viewed by all users of the organization.
-
Upcoming displays future models that will soon be available in the Model Hub for download and can be viewed by all users of the organization.
-
All users of the organization will receive a notification that a new model is ready to be downloaded.
-
Only organization administrators (OrgAdmin) can download models to the Model Hub. Once downloaded, models will be available in all tenants.
-
-
Available displays models that have been downloaded by organization administrators (OrgAdmin) to the Model Hub. These models can be viewed by all users of the organization.
-
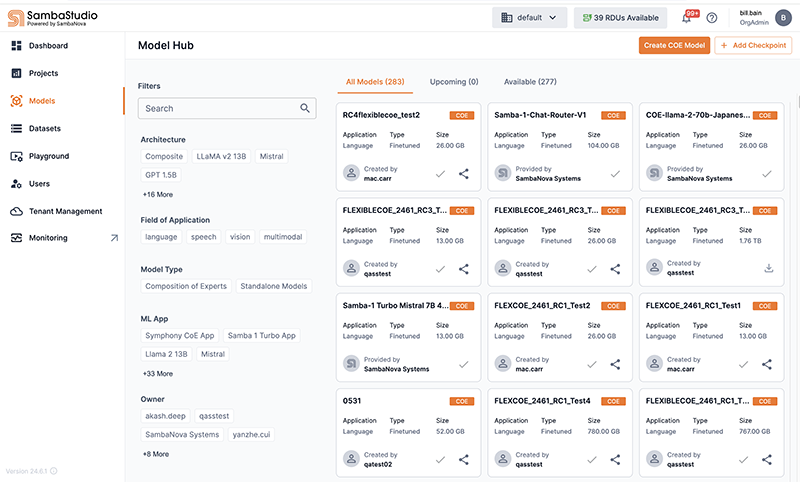
View a CoE model card
SambaStudio SN40L users can use Composition of Experts (CoE) models. CoE models are indicated by a CoE badge in their previews and model cards.
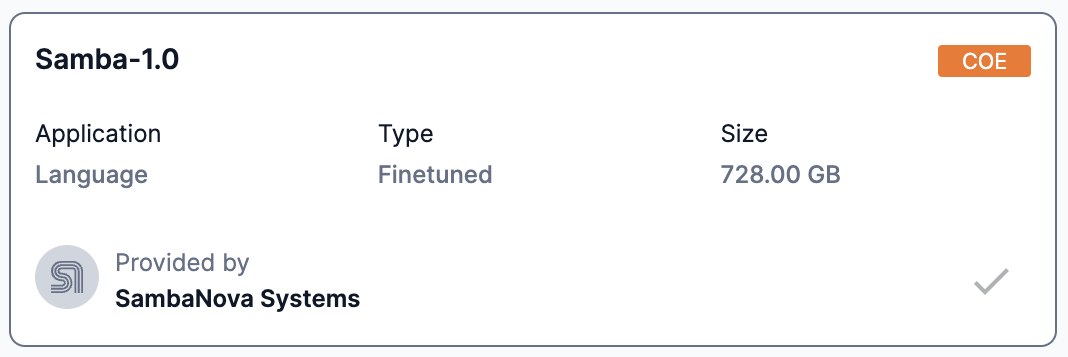
Click a CoE model card preview to view detailed information about that model including:
-
The status of the model.
-
Available designates that the model has been downloaded by organization administrators (OrgAdmin) and is ready to use.
-
Download designates that the model can be downloaded by organization administrators (OrgAdmin) to be used.
-
-
Application denotes the model’s application type of Language.
-
Type displays the CoE model type.
-
Size displays the storage size of the model.
-
Model version denotes the version of the model.
-
Owner denotes the CoE model owner.
-
Overview provides useful information about the model.
-
Experts in this Composition lists the expert models used to create the Samba-1 CoE model.
-
You can adjust the number of rows displayed and page through the list.
-
Hover over each expert to view its description.
-
-
-
The supported SambaNova hardware generation (SN40L) for each job type.
-
The minimum number of RDUs required for each job type.
-
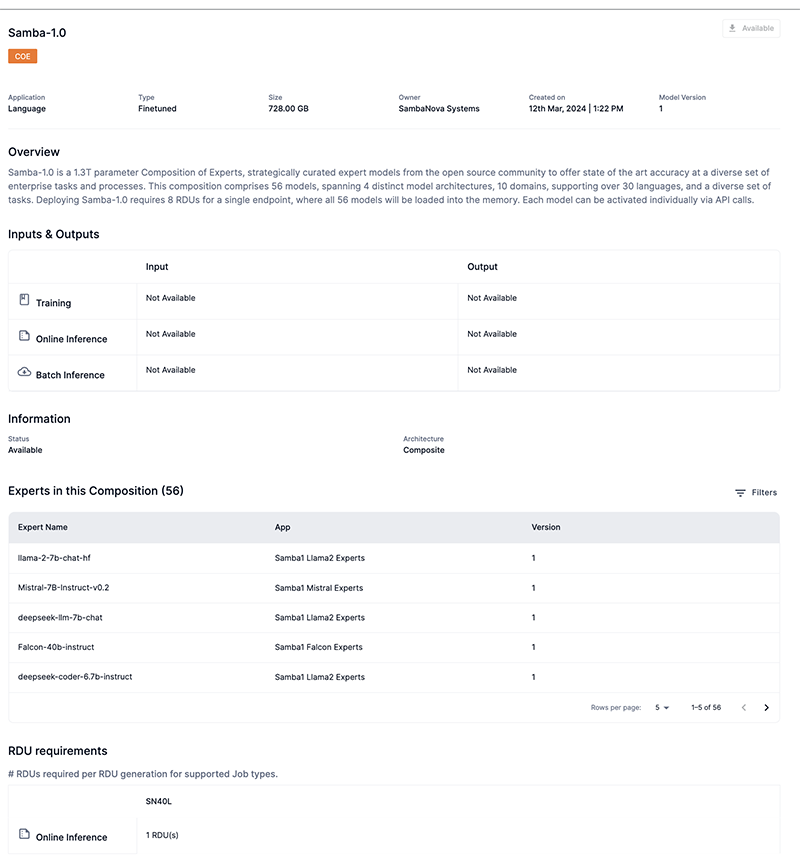
View a model card
Click a model card preview to view detailed information about that model including:
-
The status of the model.
-
Available designates that the model has been downloaded by organization administrators (OrgAdmin) and is ready to use.
-
Download designates that the model can be downloaded by organization administrators (OrgAdmin) to be used.
-
-
Application denotes the model’s application type of Speech, Language, or Vision.
-
Languages displays the languages supported by Speech or Language models.
-
Type displays the model type including Base, Finetuned, and Pretrained.
-
Size displays the storage size of the model.
-
Owner denotes the model owner.
-
Overview provides useful information about the model.
-
Dataset provides information about the training and dataset(s) used for the model.
-
Inputs & Outputs provides input/output specifics for supported tasks.
-
Information displays the status and architecture of the model.
-
-
The supported SambaNova hardware generation (SN10, SN30, SN40L) for each job type.
-
The minimum number of RDUs required for each job type.
-
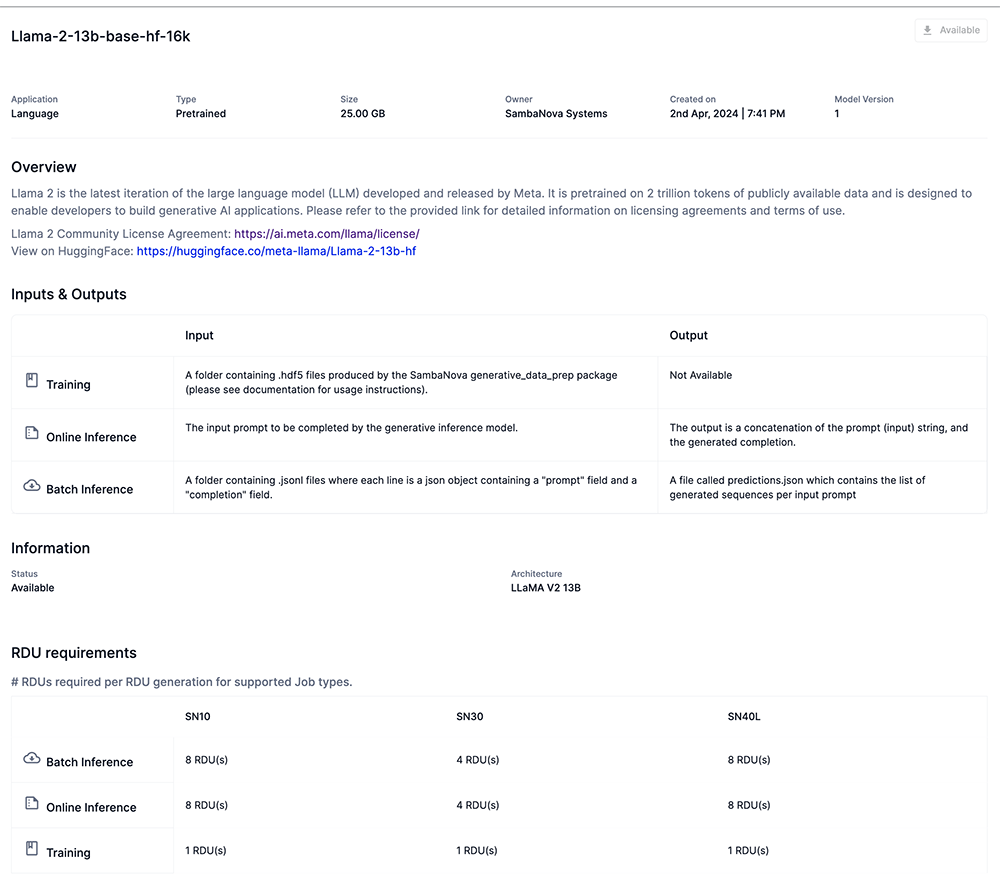
View the model list using the CLI
Similar to the GUI, the SambaNova API (snapi) provides the ability to view the repository of models (the model list) via the CLI. The example below demonstrates the snapi model list command. You can include the --verbose option to provide additional model information such as the assigned model ID.
Our examples only display the information for one model of the complete list. |
The following information is displayed for each model in the list:
-
The Name of the model.
-
The App ID of the model.
-
The Dataset information of the model.
-
The Status of the model.
-
Available designates that the model has been downloaded by organization administrators (OrgAdmin) and is ready to use.
-
AvailableToDownload designates that the model can be downloaded by organization administrators (OrgAdmin) to be used.
-
$ snapi model list
GPT13B 2k SS HAv3
============
Name : GPT13B 2k SS HAv3
App : 57f6a3c8-1f04-488a-bb39-3cfc5b4a5d7a
Dataset : { 'info': '\n'
'The starting point for this checkpoint was the GPT 13B 8k SS '
'checkpoint which had been trained on 550B pretraining tokens,\n'
'and further instruction-tuned on 39.3B tokens of instruction '
'data. We then trained this checkpoint on the following datasets:\n'
'\n'
'1. databricks-dolly-15k\n'
'2. oasst1\n'
'\n'
'We trained on this mixture for 16 epochs.\n',
'url': ''}
Status : Available
Click to view the example snapi model list --verbose command.
$ snapi model list \
--verbose
GPT13B 2k SS HAv3
============
ID : c7be342b-208b-4393-b5c2-496aa54eb917
Name : GPT13B 2k SS HAv3
Architecture : GPT 13B
Field of Application : language
Validation Loss : -
Validation Accuracy : -
App : 57f6a3c8-1f04-488a-bb39-3cfc5b4a5d7a
Dataset : { 'info': '\n'
'The starting point for this checkpoint was the GPT 13B 8k SS '
'checkpoint which had been trained on 550B pretraining tokens,\n'
'and further instruction-tuned on 39.3B tokens of instruction '
'data. We then trained this checkpoint on the following datasets:\n'
'\n'
'1. databricks-dolly-15k\n'
'2. oasst1\n'
'\n'
'We trained on this mixture for 16 epochs.\n',
'url': ''}
SambaNova Provided : True
Version : 1
Description : Pre-trained large language models excel in predicting the next word in sentences, but are not aligned for generating the correct responses for many of the common use cases, such as summarization or question answering. Human-facing applications in particular, such as for a chatbot, are a pain point. This checkpoint has been trained on human alignment data to optimize it for such applications. This checkpoint can serve two primary use cases:
1. It can be directly used for human-facing applications.
2. It can be used as a starting checkpoint for further alignment to instill further human-aligned qualities, such as politeness, helpfulness, or harmlessness. Some of its instruction-following capabilities may have been lost in the human alignment process, but it is still usable for instruction following applications.
This checkpoint is the same as the 8k SS HAv3 checkpoint, but has its positional embeddings truncated to 2048. If you expect to work with shorter sequences, 2k SS HAv3 will have slightly faster inference latency.
Please run inference with do_sample=True and a sampling temperature >= 0.7 for best results.
Created Time : 2024-01-19 14:35:42.274822 +0000 UTC
Status : Available
Steps : 0
Hyperparameters :
{'batch_predict': {}, 'deploy': {}, 'train': {}}
Size In GB : 49
Checkpoint Type : finetuned
Model IO : { 'infer': { 'input': {'description': '', 'example': ''},
'output': {'description': '', 'example': ''}},
'serve': { 'input': {'description': '', 'example': ''},
'output': { 'description': 'The output is a concatenation '
'of the prompt (input) string, '
'and the generated completion.',
'example': ''}},
'train': { 'input': {'description': '', 'example': ''},
'output': {'description': '', 'example': ''}}}
Evaluation : {}
Params : { 'invalidates_checkpoint': {'max_seq_length': 2048, 'vocab_size': 50260},
'modifiable': None}
rdu
Run snapi model list --help to display additional usage and options. |
View model information using the CLI
The example below demonstrates how to view detailed information for a specific model using the snapi model info command. You will need to specify the following:
-
The model name or ID for the --model input.
-
Run the snapi model list command and include the --verbose option to view the model IDs for each model.
-
-
The type of job to get detailed information for the --job-type input.
-
Input train for training jobs, batch_predict for batch inference jobs, or deploy for endpoints.
-
Click to view the example snapi model info command.
$ snapi model info \
--model c7be342b-208b-4393-b5c2-496aa54eb917 \
--job-type train
Model Info
============
ID : c7be342b-208b-4393-b5c2-496aa54eb917
Name : GPT13B 2k SS HAv3
Architecture : GPT 13B
Field of Application : language
Validation Loss : -
Validation Accuracy : -
App : 57f6a3c8-1f04-488a-bb39-3cfc5b4a5d7a
Dataset : { 'info': '\n'
'The starting point for this checkpoint was the GPT 13B 8k SS '
'checkpoint which had been trained on 550B pretraining tokens,\n'
'and further instruction-tuned on 39.3B tokens of instruction '
'data. We then trained this checkpoint on the following datasets:\n'
'\n'
'1. databricks-dolly-15k\n'
'2. oasst1\n'
'\n'
'We trained on this mixture for 16 epochs.\n',
'url': ''}
SambaNova Provided : True
Version : 1
Description : Pre-trained large language models excel in predicting the next word in sentences, but are not aligned for generating the correct responses for many of the common use cases, such as summarization or question answering. Human-facing applications in particular, such as for a chatbot, are a pain point. This checkpoint has been trained on human alignment data to optimize it for such applications. This checkpoint can serve two primary use cases:
1. It can be directly used for human-facing applications.
2. It can be used as a starting checkpoint for further alignment to instill further human-aligned qualities, such as politeness, helpfulness, or harmlessness. Some of its instruction-following capabilities may have been lost in the human alignment process, but it is still usable for instruction following applications.
This checkpoint is the same as the 8k SS HAv3 checkpoint, but has its positional embeddings truncated to 2048. If you expect to work with shorter sequences, 2k SS HAv3 will have slightly faster inference latency.
Please run inference with do_sample=True and a sampling temperature >= 0.7 for best results.
Created Time : 2024-01-19 14:35:42.274822 +0000 UTC
Status : Available
Steps : 0
Hyperparameters :
{ 'batch_predict': {},
'deploy': {},
'train': { 'sn10': { 'imageVariants': [],
'imageVersion': '3.0.0-20231218',
'jobTypes': ['compile', 'train'],
'runtimeVersion': '5.3.0',
'sockets': 2,
'supports_data_parallel': True,
'user_params': [ { 'CONSTRAINTS': { 'ge': '',
'gt': '',
'le': '',
'lt': '',
'values': [ 'true',
'false']},
'DATATYPE': 'bool',
'DESCRIPTION': 'whether or '
'not to do '
'final '
'evaluation',
'FIELD_NAME': 'do_eval',
'MESSAGE': '',
'TASK_TYPE': ['train'],
'TYPE_SPECIFIC_SETTINGS': { 'train': { 'DEFAULT': 'true',
'USER_MODIFIABLE': True}},
'VARIANT_SELECTION': False},
{ 'CONSTRAINTS': { 'ge': '1',
'gt': '',
'le': '',
'lt': '',
'values': [ ]},
'DATATYPE': 'int',
'DESCRIPTION': 'Period of '
'evaluating '
'the model '
'in number '
'of '
'training '
'steps. '
'This '
'parameter '
'is only '
'effective '
'when '
'evaluation_strategy '
'is set to '
"'steps'.",
'FIELD_NAME': 'eval_steps',
'MESSAGE': '',
'TASK_TYPE': ['train'],
'TYPE_SPECIFIC_SETTINGS': { 'train': { 'DEFAULT': '50',
'USER_MODIFIABLE': True}},
'VARIANT_SELECTION': False},
{ 'CONSTRAINTS': { 'ge': '',
'gt': '',
'le': '',
'lt': '',
'values': [ 'no',
'steps',
'epoch']},
'DATATYPE': 'str',
'DESCRIPTION': 'Strategy '
'to '
'validate '
'the model '
'during '
'training',
'FIELD_NAME': 'evaluation_strategy',
'MESSAGE': '',
'TASK_TYPE': ['train'],
'TYPE_SPECIFIC_SETTINGS': { 'train': { 'DEFAULT': 'steps',
'USER_MODIFIABLE': True}},
'VARIANT_SELECTION': False},
{ 'CONSTRAINTS': { 'ge': '0',
'gt': '',
'le': '',
'lt': '',
'values': [ ]},
'DATATYPE': 'float',
'DESCRIPTION': 'learning '
'rate to '
'use in '
'optimizer',
'FIELD_NAME': 'learning_rate',
'MESSAGE': '',
'TASK_TYPE': ['train'],
'TYPE_SPECIFIC_SETTINGS': { 'train': { 'DEFAULT': '7.5e-06',
'USER_MODIFIABLE': True}},
'VARIANT_SELECTION': False},
{ 'CONSTRAINTS': { 'ge': '1',
'gt': '',
'le': '',
'lt': '',
'values': [ ]},
'DATATYPE': 'int',
'DESCRIPTION': 'Period of '
'logging '
'training '
'loss in '
'number of '
'training '
'steps',
'FIELD_NAME': 'logging_steps',
'MESSAGE': '',
'TASK_TYPE': ['train'],
'TYPE_SPECIFIC_SETTINGS': { 'train': { 'DEFAULT': '10',
'USER_MODIFIABLE': True}},
'VARIANT_SELECTION': False},
{ 'CONSTRAINTS': { 'ge': '',
'gt': '',
'le': '',
'lt': '',
'values': [ 'polynomial_decay_schedule_with_warmup',
'cosine_schedule_with_warmup',
'fixed_lr']},
'DATATYPE': 'str',
'DESCRIPTION': 'Type of '
'learning '
'rate '
'scheduler '
'to use',
'FIELD_NAME': 'lr_schedule',
'MESSAGE': '',
'TASK_TYPE': ['train'],
'TYPE_SPECIFIC_SETTINGS': { 'train': { 'DEFAULT': 'cosine_schedule_with_warmup',
'USER_MODIFIABLE': True}},
'VARIANT_SELECTION': False},
{ 'CONSTRAINTS': { 'ge': '',
'gt': '',
'le': '',
'lt': '',
'values': [ '2048',
'8192']},
'DATATYPE': 'int',
'DESCRIPTION': 'Sequence '
'length to '
'pad or '
'truncate '
'the '
'dataset',
'FIELD_NAME': 'max_seq_length',
'MESSAGE': '',
'TASK_TYPE': [ 'compile',
'infer',
'serve',
'train'],
'TYPE_SPECIFIC_SETTINGS': { 'train': { 'DEFAULT': '2048',
'USER_MODIFIABLE': False}},
'VARIANT_SELECTION': False},
{ 'CONSTRAINTS': { 'ge': '1',
'gt': '',
'le': '',
'lt': '',
'values': [ ]},
'DATATYPE': 'int',
'DESCRIPTION': 'number of '
'iterations '
'to run',
'FIELD_NAME': 'num_iterations',
'MESSAGE': '',
'TASK_TYPE': ['train'],
'TYPE_SPECIFIC_SETTINGS': { 'train': { 'DEFAULT': '100',
'USER_MODIFIABLE': True}},
'VARIANT_SELECTION': False},
{ 'CONSTRAINTS': { 'ge': '0',
'gt': '',
'le': '',
'lt': '',
'values': [ ]},
'DATATYPE': 'float',
'DESCRIPTION': 'Loss scale '
'for prompt '
'tokens',
'FIELD_NAME': 'prompt_loss_weight',
'MESSAGE': '',
'TASK_TYPE': ['train'],
'TYPE_SPECIFIC_SETTINGS': { 'train': { 'DEFAULT': '0.1',
'USER_MODIFIABLE': True}},
'VARIANT_SELECTION': False},
{ 'CONSTRAINTS': { 'ge': '',
'gt': '',
'le': '',
'lt': '',
'values': [ 'true',
'false']},
'DATATYPE': 'bool',
'DESCRIPTION': 'Whether to '
'save the '
'optimizer '
'state when '
'saving a '
'checkpoint',
'FIELD_NAME': 'save_optimizer_state',
'MESSAGE': '',
'TASK_TYPE': ['train'],
'TYPE_SPECIFIC_SETTINGS': { 'train': { 'DEFAULT': 'true',
'USER_MODIFIABLE': True}},
'VARIANT_SELECTION': False},
{ 'CONSTRAINTS': { 'ge': '1',
'gt': '',
'le': '',
'lt': '',
'values': [ ]},
'DATATYPE': 'int',
'DESCRIPTION': 'Period of '
'saving the '
'model '
'checkpoints '
'in number '
'of '
'training '
'steps',
'FIELD_NAME': 'save_steps',
'MESSAGE': '',
'TASK_TYPE': ['train'],
'TYPE_SPECIFIC_SETTINGS': { 'train': { 'DEFAULT': '50',
'USER_MODIFIABLE': True}},
'VARIANT_SELECTION': False},
{ 'CONSTRAINTS': { 'ge': '',
'gt': '',
'le': '',
'lt': '',
'values': [ 'true',
'false']},
'DATATYPE': 'bool',
'DESCRIPTION': 'whether or '
'not to '
'skip the '
'checkpoint',
'FIELD_NAME': 'skip_checkpoint',
'MESSAGE': '',
'TASK_TYPE': ['train'],
'TYPE_SPECIFIC_SETTINGS': { 'train': { 'DEFAULT': 'false',
'USER_MODIFIABLE': True}},
'VARIANT_SELECTION': False},
{ 'CONSTRAINTS': { 'ge': '0',
'gt': '',
'le': '',
'lt': '',
'values': [ ]},
'DATATYPE': 'float',
'DESCRIPTION': 'Subsample '
'for the '
'evaluation '
'dataset',
'FIELD_NAME': 'subsample_eval',
'MESSAGE': '',
'TASK_TYPE': ['train'],
'TYPE_SPECIFIC_SETTINGS': { 'train': { 'DEFAULT': '0.01',
'USER_MODIFIABLE': True}},
'VARIANT_SELECTION': False},
{ 'CONSTRAINTS': { 'ge': '1',
'gt': '',
'le': '',
'lt': '',
'values': [ ]},
'DATATYPE': 'int',
'DESCRIPTION': 'Random '
'seed to '
'use for '
'the '
'subsample '
'evaluation',
'FIELD_NAME': 'subsample_eval_seed',
'MESSAGE': '',
'TASK_TYPE': ['train'],
'TYPE_SPECIFIC_SETTINGS': { 'train': { 'DEFAULT': '123',
'USER_MODIFIABLE': True}},
'VARIANT_SELECTION': False},
{ 'CONSTRAINTS': { 'ge': '',
'gt': '',
'le': '',
'lt': '',
'values': [ 'true',
'false']},
'DATATYPE': 'bool',
'DESCRIPTION': 'Whether to '
'use '
'token_type_ids '
'to compute '
'loss',
'FIELD_NAME': 'use_token_type_ids',
'MESSAGE': '',
'TASK_TYPE': ['train'],
'TYPE_SPECIFIC_SETTINGS': { 'train': { 'DEFAULT': 'true',
'USER_MODIFIABLE': True}},
'VARIANT_SELECTION': False},
{ 'CONSTRAINTS': { 'ge': '',
'gt': '',
'le': '',
'lt': '',
'values': [ '50260',
'307200']},
'DATATYPE': 'int',
'DESCRIPTION': 'Maximum '
'size of '
'vocabulary',
'FIELD_NAME': 'vocab_size',
'MESSAGE': '',
'TASK_TYPE': [ 'compile',
'infer',
'serve',
'train'],
'TYPE_SPECIFIC_SETTINGS': { 'train': { 'DEFAULT': '50260',
'USER_MODIFIABLE': False}},
'VARIANT_SELECTION': False},
{ 'CONSTRAINTS': { 'ge': '0',
'gt': '',
'le': '',
'lt': '',
'values': [ ]},
'DATATYPE': 'int',
'DESCRIPTION': 'warmup '
'steps to '
'use in '
'learning '
'rate '
'scheduler '
'in '
'optimizer',
'FIELD_NAME': 'warmup_steps',
'MESSAGE': '',
'TASK_TYPE': ['train'],
'TYPE_SPECIFIC_SETTINGS': { 'train': { 'DEFAULT': '0',
'USER_MODIFIABLE': True}},
'VARIANT_SELECTION': False},
{ 'CONSTRAINTS': { 'ge': '0',
'gt': '',
'le': '',
'lt': '',
'values': [ ]},
'DATATYPE': 'float',
'DESCRIPTION': 'weight '
'decay rate '
'to use in '
'optimizer',
'FIELD_NAME': 'weight_decay',
'MESSAGE': '',
'TASK_TYPE': [ 'infer',
'serve',
'train'],
'TYPE_SPECIFIC_SETTINGS': { 'train': { 'DEFAULT': '0.1',
'USER_MODIFIABLE': True}},
'VARIANT_SELECTION': False}],
'variantSetVersion': ''}}}
Size In GB : 49
Checkpoint Type : finetuned
Model IO : { 'infer': { 'input': {'description': '', 'example': ''},
'output': {'description': '', 'example': ''}},
'serve': { 'input': {'description': '', 'example': ''},
'output': { 'description': 'The output is a concatenation '
'of the prompt (input) string, '
'and the generated completion.',
'example': ''}},
'train': { 'input': {'description': '', 'example': ''},
'output': {'description': '', 'example': ''}}}
Evaluation : {}
Params : { 'invalidates_checkpoint': {'max_seq_length': 2048, 'vocab_size': 50260},
'modifiable': None}
rdu
Create ASR pipelines
SambaStudio allows you to create new ASR pipelines that utilize your trained Hubert ASR models. You can use your trained Hubert ASR models to create a new ASR pipeline with diarization (Diarization ASR Pipeline) or without diarization (ASR Pipeline). The process is the same for both workflows. Follow the steps below to create either a new ASR Pipeline or a new Diarization ASR Pipeline.
-
Click Create new pipeline from the kebob menu (three vertical dots) drop-down of either the model card preview or the detailed model card.
Figure 6. Create new pipeline from a model card previewFigure 7. Create new pipeline from a detailed model card-
The Create pipeline model window will open.
-
-
In the Create pipeline model window, select your trained Hubert ASR model to replace the default Hubert_ASR model from the drop-down.
-
We used our trained Hubert_ASR_finetuned_02 model in the example below.
-
-
Enter a new name into the Pipeline model name field.
-
Select either finetuned or pretrained from the Model type drop-down.
-
The Share settings drop-down provides options for which tenant to share your new pipeline model.
-
Share with <current-tenant> allows the new model to be shared with the current tenant you are using, identified by its name in the drop-down.
-
Share with all tenants allows the new model to be shared across all tenants.
-
-
Click Save model to save the new ASR pipeline to the Model Hub.
Figure 8. Create pipeline model window
Download models using the GUI
SambaStudio provides the ability for organization administrators (OrgAdmin) to download models to the Model Hub. This allows SambaNova created models to be downloaded and used when new models are released. A model available for download will display a download icon in the associated model cards panel and its model card.
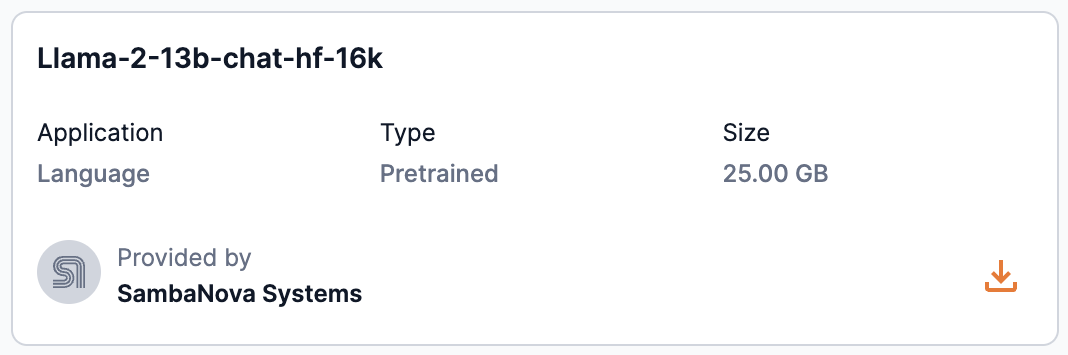

Add models using the GUI
SambaStudio allows you to add a model from a training job or from local storage. From the Model Hub window, click Add checkpoint to open the Add a checkpoint to Model Hub box to start the process.

Add a checkpoint from a training job using the GUI
Follow the steps below to add a checkpoint from a training job using the SambaStudio GUI.
-
From the Model Hub window, click Add checkpoint. The Add a checkpoint to Model Hub box will open.
-
Select Training job for the Source.
-
From the Select project drop-down, select the project that contains checkpoint.
-
Enter a name for the new model-checkpoint in the Model name field.
-
From the Select job drop-down, select the job that contains the checkpoint.
-
The Select checkpoint drop-down opens a list of available checkpoints from the specified job. Select the model-checkpoint you wish to add from the list.
-
Select finetuned or pretrained from the Select model type drop-down.
-
Click Add checkpoint to Model Hub to confirm adding your selected checkpoint and return to the Model Hub. Click Cancel to close the box and return to the Model Hub.
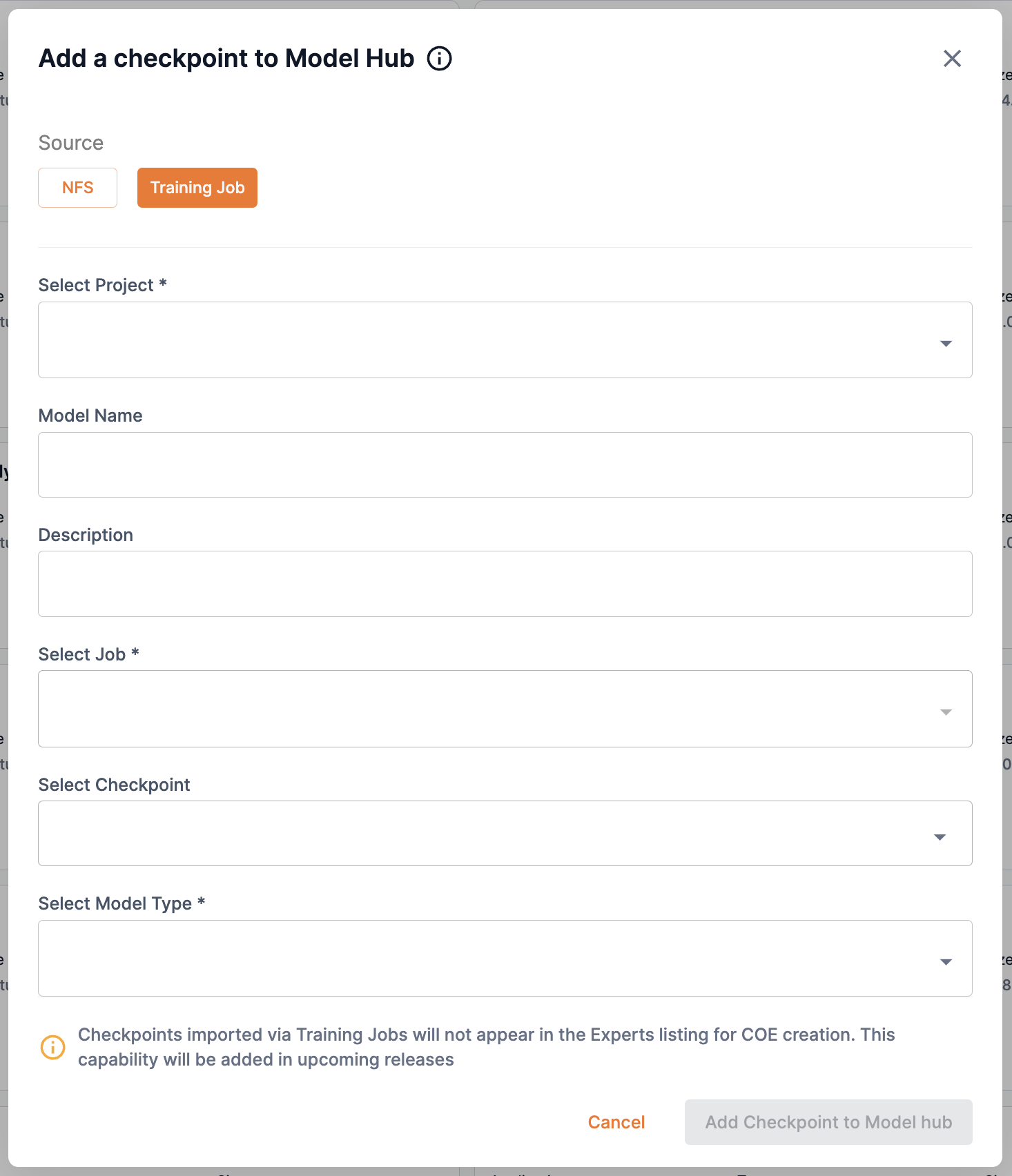
Add a checkpoint from storage using the GUI
Adding a checkpoint from storage is a beta feature of SambaStudio. As a beta release, this feature provides an initial procedure that will become full-featured in subsequent SambaStudio releases. This initial beta release requires the following:
|
SambaStudio allows you to add a checkpoint as a new model to your SambaStudio environment NFS. This enables you to add checkpoints to SambaStudio that are model architecture compatible, as well as being compatible with at least one supported configuration of SambaStudio. Adding a checkpoint to the Model Hub is a four step process as described below.
Step 1: Details
-
From the Add a checkpoint to Model Hub box, select NFS as the Source.
-
Append the model path, provided by your administrator, from your NFS location in the Import model path field. An example path would be
byoc/Llama-2-7b-chat-hf-bf16
. -
Enter a unique name for the new model-checkpoint in the Import model name field.
-
From the Select ML App drop-down, choose the ML App to which the model-checkpoint belongs.
-
Click Next to submit your details and proceed to Step 2: Validation.
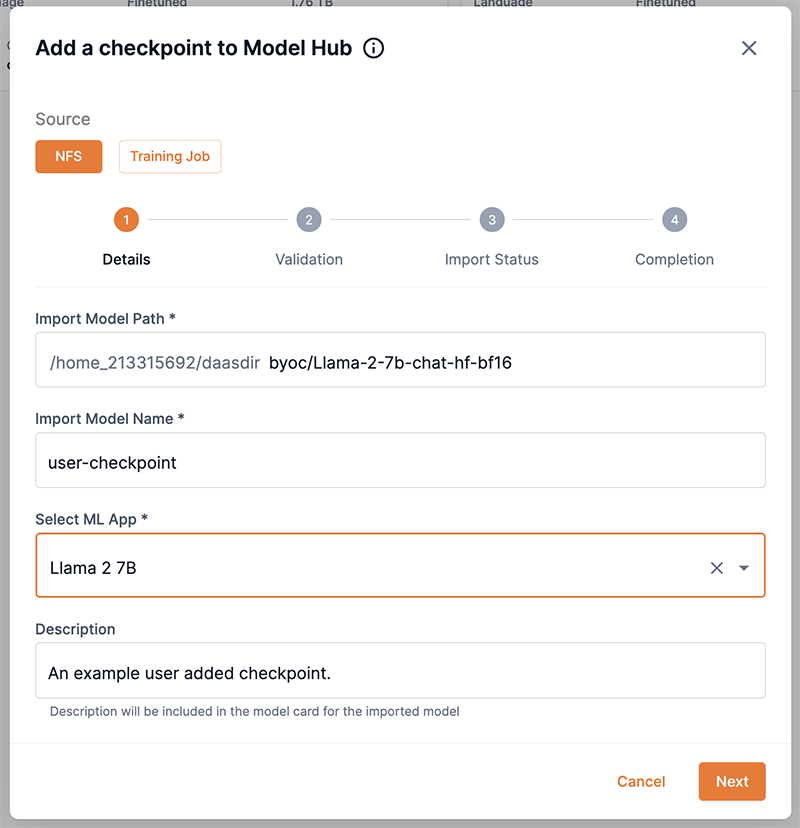
Step 2: Validation
-
Enter the publisher’s name of the checkpoint in the Publisher name field. Unknown is provided by default.
-
Ensure the Model architecture field is correct, otherwise input the architecture of the model.
-
Input the model parameter count of your checkpoint into the Parameter count field.
-
Ensure the checkpoint’s sequence length is correct, otherwise enter it in the Sequence length field.
-
Ensure the checkpoint’s vocab size is correct, otherwise enter it into the Vocab size field.
-
Click Run check to initiate the review of inputted information.
Figure 14. Step 2: Validation run check -
The run check results will be displayed with pass or warning statements.
-
Inference is not supported designates the ability to deploy an inference checkpoint as a non-CoE model.
-
Training supported designates that the checkpoint supports training.
-
CoE model supported designates the ability to deploy an inference checkpoint as a CoE model.
-
If one warning statement displays, such as Inference is not supported, you will receive the configuration is not fully supported statement. You may still Proceed to Step 3: Import status. You may also click Back to return to Step 1: Details and update your checkpoint’s information. Please see the guidelines for addressing issues you might encounter.
Figure 15. Step 2: Validation back
-
-
Click Proceed to go to Step 3: Import status.
Figure 16. Step 2: Validation proceed
Step 3: Import status
The importing of the model will begin after you have clicked Proceed in Step 2: Validation. The Add a checkpoint to Model Hub box will display notifications describing the import process and status.
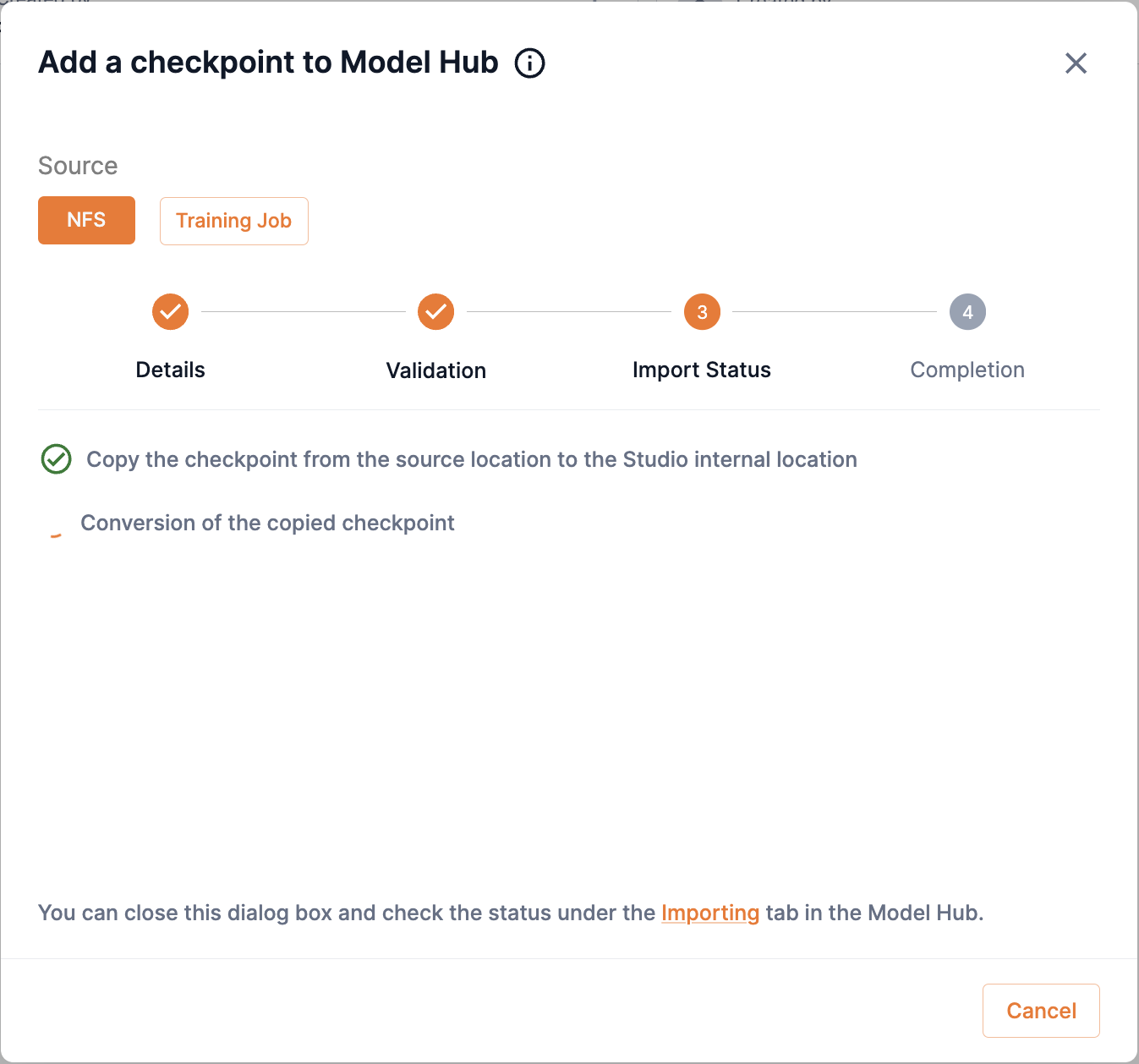
Step 4: Completion
The Completion screen will display confirming that your import was successful or will describe any issues. Note that models are private by default. Please adjust the model share settings share your model with other users and tenants.
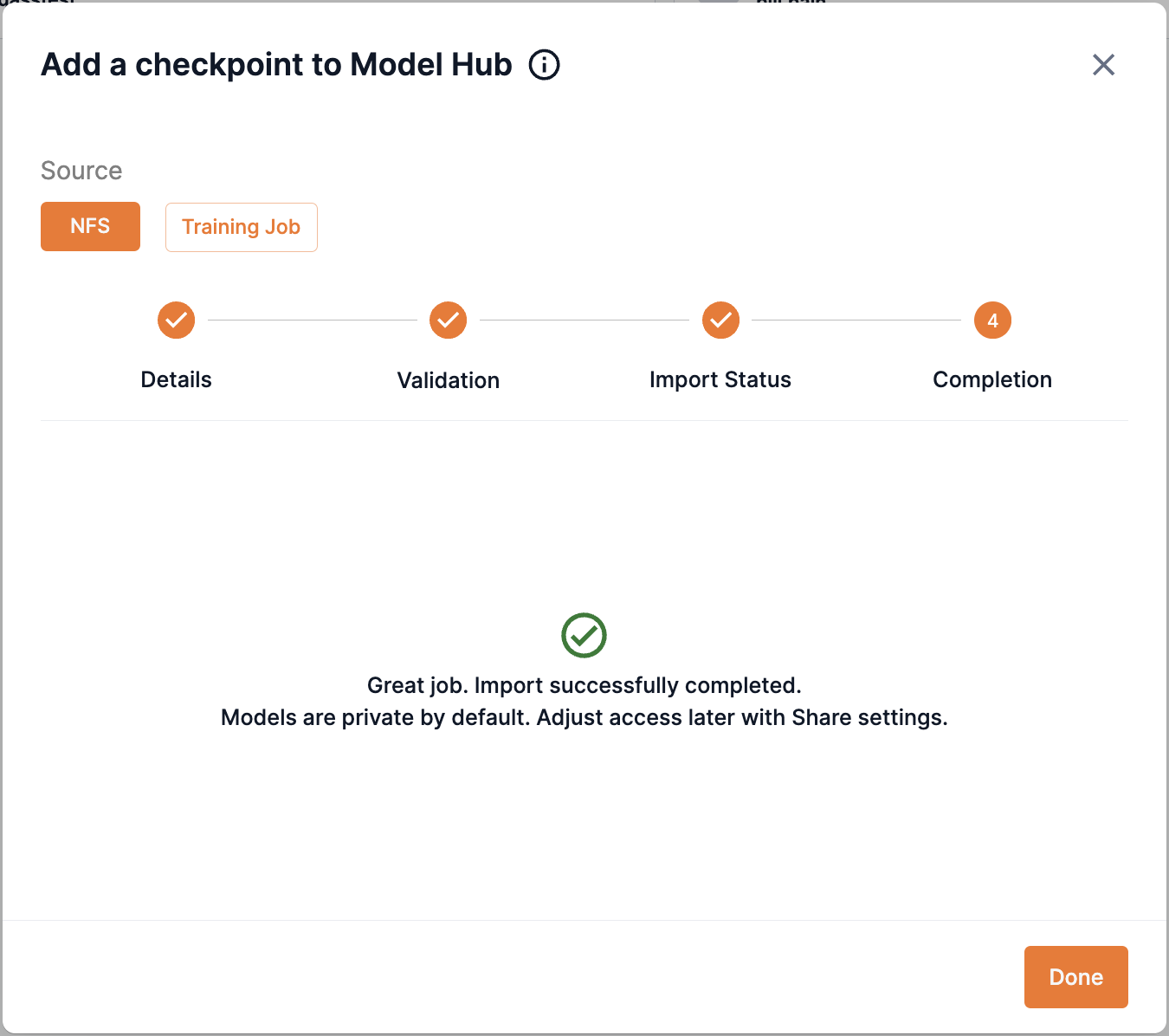
Insufficient storage message
If the required amount of storage space is not available to add the checkpoint, the Insufficient storage message will display describing the Available space and the Required space to import the model. You will need to free up storage space or contact your administrator. Please choose one of the following options.
-
Click Cancel to stop the add a checkpoint process. Please free up storage space and then restart the add a checkpoint to the Model Hub process.
-
Click Add checkpoint to Model Hub to proceed and add the checkpoint. Please free up storage space, otherwise the add a checkpoint to the Model Hub process will fail.
A minimum of 10 minutes is required after sufficient storage space has been cleared before the checkpoint will start successfully saving to the Model Hub. |
Why my add checkpoint validation is failing
This section describes guidelines for addressing issues you might encounter when adding a checkpoint from storage using the GUI.
Input type and values
The table below describes the input types and accepted values to use when adding a checkpoint from storage using the GUI.
Attribute | Type | Description |
---|---|---|
Parameter Count |
String |
The Parameter Count input value as described in List of ML App supported values. Input values must be formatted using a numeral followed by a lowercase character, such as 7b or 13b. |
Sequence Length |
String |
The supported Max Sequence Length value as described in List of ML App supported values. |
Original Vocab Size |
Number |
The supported Vocab Size value as described in List of ML App supported values. |
List of ML App supported values
The table below describes the supported values for each ML App when adding a checkpoint from storage using the GUI.
Any combination of the values in the table are supported. |
ML App Name | Parameter Count | Sequence Length | Original Vocab Size |
---|---|---|---|
Samba1 Llama2 Experts |
7b 7b 70b 13b 1.3b 6.7b 33b 13b 70b 7b 7b 67b |
4096 8192 4096 8192 16384 16384 16384 4096 4096 4096 4096 4096 |
57344 57344 32000 57344 57344 57344 57344 57344 57344 102400 102400 102400 |
Samba1 Llama3 Experts |
70b 8b |
4096 4096 |
128256 128256 |
Samba1 Mistral Experts |
7b 7b 7b 7b 7b 7b |
32768 32768 32768 4096 4096 4096 |
32000 32000 32000 32000 57344 57344 |
Samba1 Gemma Experts |
7b |
8192 |
256000 |
Samba1 Bloom Experts |
176b 176b 176b 176b |
8192 8192 2048 2048 |
250880 250880 250880 250880 |
Samba1 Falcon Experts |
40b |
2048 |
65024 |
DeepSeek Coder |
6.7b |
4096 |
32256 |
Mistral |
7b 7b |
8192 32768 |
32000 32000 |
Unsupported ML Apps
The ML Apps listed below are currently unsupported for adding a checkpoint from storage using the GUI.
-
ASR With Diarization
-
ASR Without Diarization
-
CoE NonMerge 20experts inference
-
Generative Tuning 1.5B
-
Generative Tuning 13B
-
Deepseek 6.7B single socket
-
Llama 2 70B
-
Llama 2 13b 8-socket
-
Molle 20experts tp8 inference
-
Image Classification
-
Molle 150experts tp8 inference
-
Named Entity Recognition
-
Reranking
-
Symphony CoE App
-
Samba 1 Turbo App
-
Sentence Detection
-
Simple Text Classifier
-
Speaker Diarization
-
Speaker Verification
-
Speech Recognition
-
DePlot
-
Dialog Act Classification
-
CLIP
Import models using the CLI
Use the snapi model import command to import a model to the model list. The example below demonstrates how to import a pretrained model from local storage and have it start at step 20. You will need to specify the following:
-
The Model ID for the --model-id input.
-
Provide the relative path to the model from your NFS location for the --import-path input.
-
A name for your model for the --import-model-name input.
$ snapi model import \
--model-id '97ea373f-498e-4ede-822e-a34a88693a09' \
--import-path 'user1/checkpoints/subpath' \
--import-model-name 'gt_model_imported' \
--storage-type 'Local' \
--steps 20
Run snapi model import --help to display additional usage and options. |
Model share settings
Providing access to models within SambaStudio is accomplished by assigning a share role in the Share settings window. Access to models can be assigned by the owner/creator of the model (a User), tenant administrators (TenantAdmin) to models within their tenant, and organization administrators (OrgAdmin) to models across all tenants.
SambaNova owned models cannot have their share settings assigned. |
Click the share icon in the model preview or Share in the top of the model card to access the Share settings window.
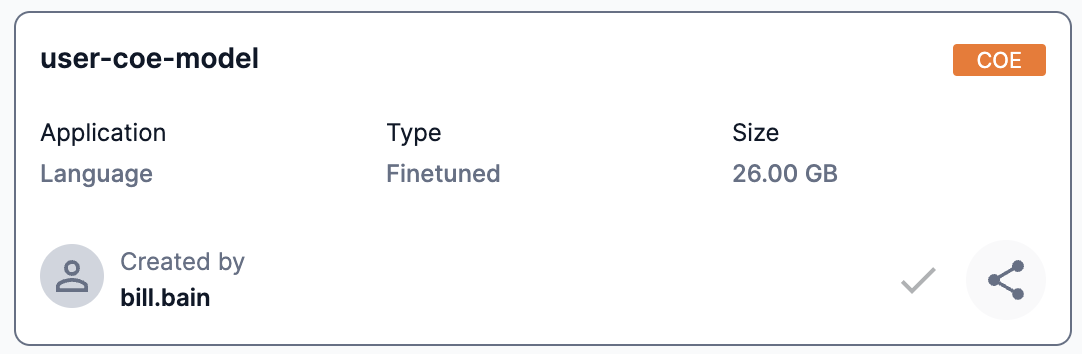

The Share settings window is divided into two sections, General access and Share with specific users. Slide the toggle to the right to enable and define share settings for either section. This allows model access to be defined and regulated based on the criteria described below.
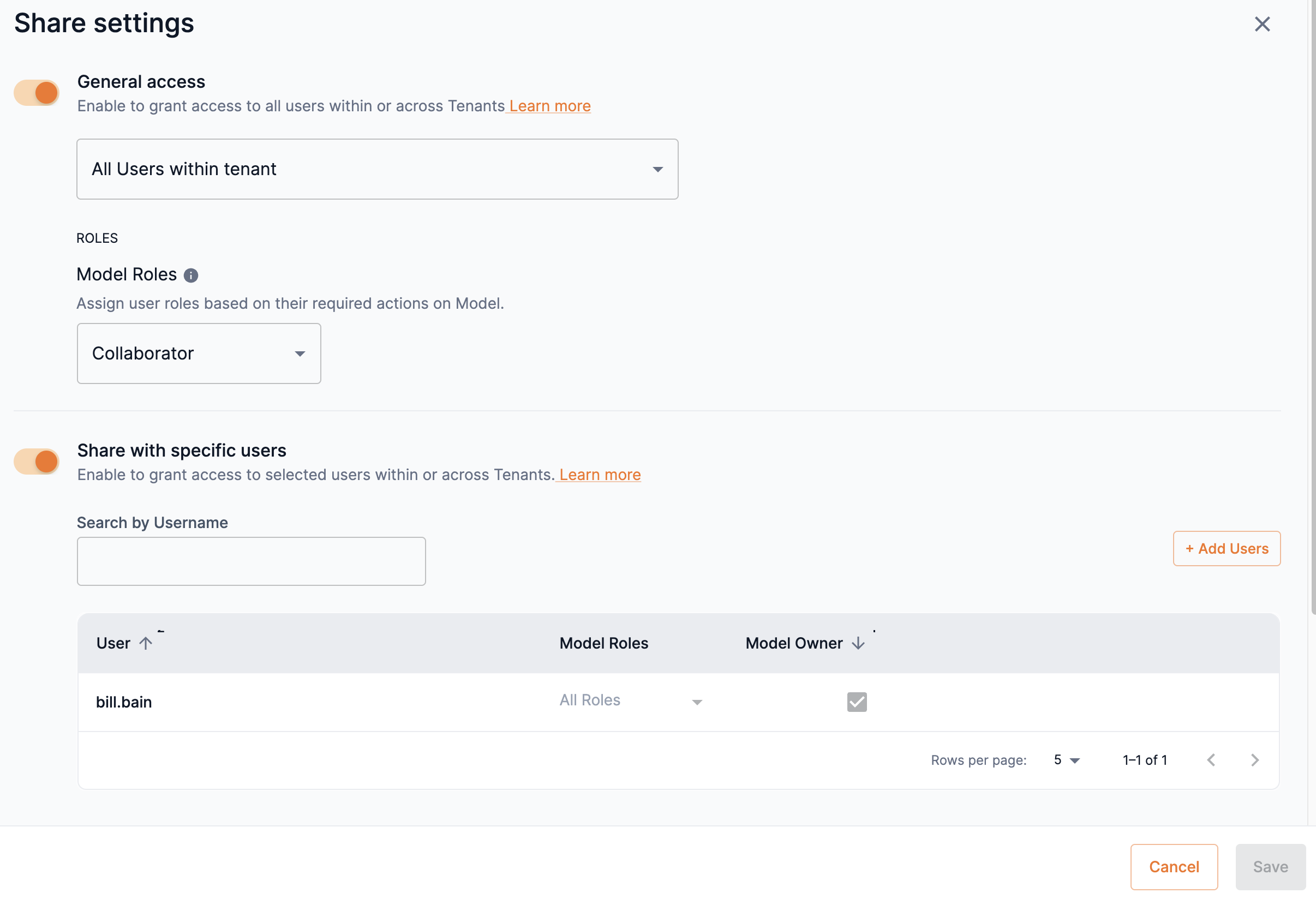
General access
You can provide access to a model based on the users in tenants. Once you have defined the general access to your model based on tenants, you can set access to a model based on the share roles of Viewer or Collaborator.
- Viewer
-
A Viewer has the most restrictive access to SambaStudio artifacts. Viewers are only able to consume and view artifacts assigned to them. Viewers cannot take actions that affect the functioning of an artifact assigned to them.
- Collaborator
-
Collaborators have more access to artifacts than Viewers. A Collaborator can edit and manipulate the information of artifacts assigned to them. As such, a Collaborator can affect the functioning of an artifact assigned to them.
Hover over the info icon to view the actions available for Viewer and Collaborator share roles of models. ![]() Figure 22. Model share roles actions
|
-
Slide the toggle to the right to enable and define access to the model based on tenants and their defined users.
-
From the drop-down, select one of the following options:
-
All users within tenant: This allows all users in the same tenant as the model to access it.
-
All users across tenants: This allows all users across all tenants in the platform to access the model.
Figure 23. Model tenant access drop-down
-
-
Select an option of Viewer or Collaborator from the Model roles drop-down to set the access to it.
-
You cannot set the option of No access to models. An information warning will appear stating that at least one role (Viewer or Collaborator) is required.
Figure 24. Model roles drop-down
-
-
Click Save at the bottom of the Share settings window to update your General access share settings.
Figure 25. Share settings save
Disable general access
-
To disable general access to a model in all tenants throughout the platform, slide the General access toggle to the left.
Figure 26. Disable general access -
Click Save at the bottom of the Share settings window to update your General access share settings.
Figure 27. Share settings save
Share with specific users
Specific users can be set as Viewer or Collaborator and allowed access to a model based on the rights of each share role. Follow the steps below to send an invitation to users and set their share role access.
OrgAdmin and TenantAdmin user roles have access to models based on their rights and cannot be assigned a different share role. |
-
Slide the Share with specific users toggle to the right to enable and define user access for the model.
-
Click Add users to open the Add new users box.
-
From the Usernames drop-down, select the user(s) for which you wish to share your model. You can type an input to locate the user. Multiple usernames can be selected.
-
You can remove a user added to the drop-down by clicking the X next to their name or by setting the cursor and pressing delete.
-
-
Select an option of Viewer or Collaborator from the Model roles drop-down to set the user’s access to it.
-
Hover over the info icon to view the actions available for Viewer and Collaborator share roles of models.
-
You cannot set the option of No access to models.
-
-
Click Add users to close the Add new users box.
Figure 28. Add new user -
You can assign a user as an owner of the model by selecting the box in the Model owner column.
-
An owner inherits the actions of all roles. Setting a user as an owner overrides previous role settings.
Figure 29. Assign user as model owner
-
-
Click Save at the bottom of the Share settings window to send the invitation to your selected users and update your share settings.
Figure 30. Share settings save
Remove users
You can remove a user that you have shared your model with for all users or for specific users.
- Remove sharing for all users
-
-
To remove share access to your model for all users, slide the Share with specific users toggle to the left to disable sharing.
Figure 31. Disable share with specific users toggle -
Click Save at the bottom of the Share settings window to update your share settings.
Figure 32. Share settings save
-
- Remove sharing for a specific user
-
-
From the Share with specific users list, click the trash icon next to the user you wish to stop sharing your model.
Figure 33. Remove user from share list -
Click Save at the bottom of the Share settings window to update your share settings.
Figure 34. Share settings save
-
Export models
SambaStudio allows users to export any checkpoint they have published to the Model Hub.
SambaNova owned models cannot be exported from the platform. |
Export a checkpoint using the GUI
Follow the steps below to export a checkpoint using the SambaStudio GUI.
-
Select your model to view its model card.
-
In the model card, click Export to the right of the model name. The Export model box will open.
-
Click Continue. The Export model box will display the source path to the exported model’s checkpoint. Copy the path displayed in Exported model path.
-
Click Done to complete the export process.
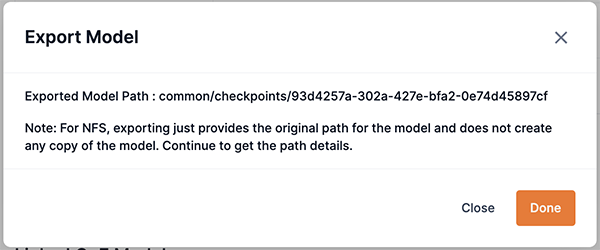
Export using the CLI
Use the snapi model export command to export a model as demonstrated below. You will need to provide the Model ID for the --model-id input.
$ snapi model export \
--model-id '97ea373f-498e-4ede-822e-a34a88693a09' \
--storage-type 'Local'
Run snapi model export --help to display additional usage and options. |
Use the snapi model list-exported command to view a list of exported models. The help command below displays the usage and options for snapi model list-exported --help.
$ snapi model list-exported --help
Usage: snapi model export [OPTIONS]
Export the model
╭─ Options ────────────────────────────────────────────────────────────────────╮
│ --file TEXT │
│ * --model-id -m TEXT Model ID [default: None] [required] │
│ --storage-type -s TEXT Supported storage type for export is │
│ 'Local' │
│ [default: Local] │
│ --help Show this message and exit. │
╰──────────────────────────────────────────────────────────────────────────────╯
Delete models
SambaStudio allows you to delete your models from the Model Hub.
SambaNova owned models cannot be deleted from the platform. |
Delete a model using the GUI
Follow the steps below to delete a model using the SambaStudio GUI.
-
Select your model to view its model card.
-
In the model card, click the trash icon to the right of the model name. The Delete model box will open. A warning message will display informing you that you are about to delete a model.
-
Click Yes to confirm that you want to delete the model.
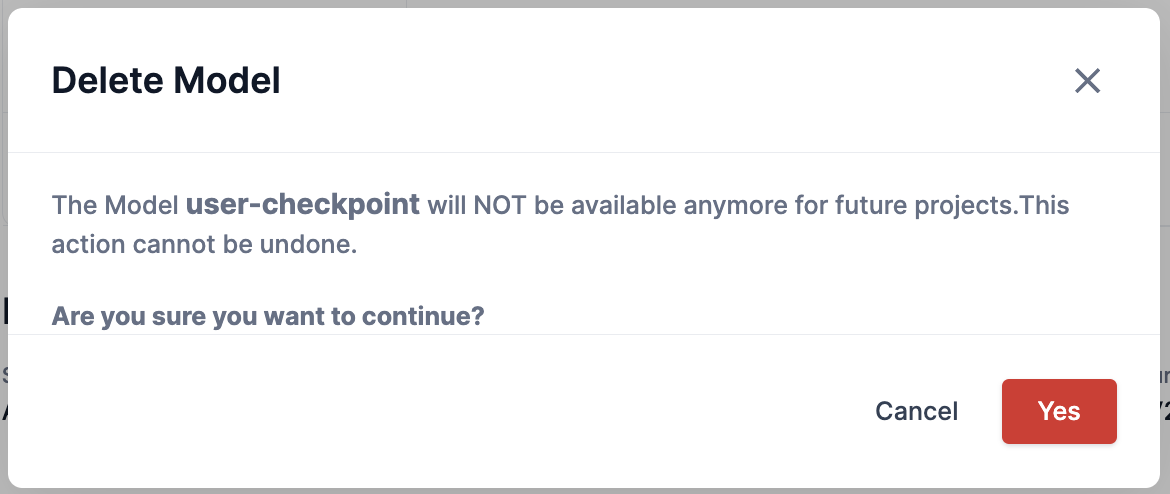
Delete a model using the CLI
Use the snapi model remove command to remove and delete a model form the Model Hub.
The help command below displays the usage and options for snapi model remove --help.
$ snapi model remove --help
Usage: snapi model remove [OPTIONS]
Remove the model
Note: For NFS, the model is not copied as a part of export. Deleting the model deletes the contents of the original model.
╭─ Options ────────────────────────────────────────────────────────────────────────────────────────────────────────────────╮
│ --file TEXT │
│ * --model -m TEXT Model Name or ID [default: None] [required] │
│ --help Show this message and exit. │
╰──────────────────────────────────────────────────────────────────────────────────────────────────────────────────────────╯
Delete an exported model using the CLI
Use the snapi model delete-exported command to delete an exported model as demonstrated below.
$ snapi model delete-exported \
--model-id '97ea373f-498e-4ede-822e-a34a88693a09' \
--model-activity-id '9abbec28-c1cf-11ed-afa1-0242ac120002'
Run snapi model delete-exported --help to display additional usage and options. |